时间序列模型
- 网络time series modeling;time series model;ARMA
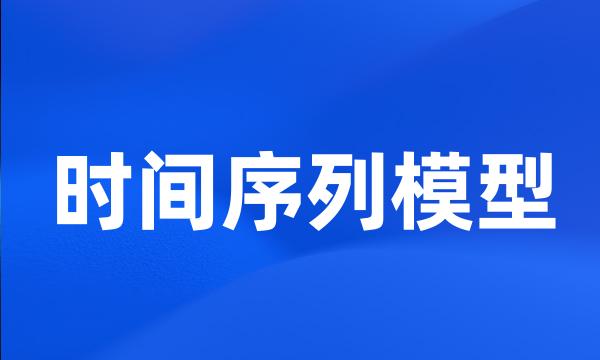
-
时间序列模型Back算子多项式的可逆性
The Invertibility of the Polynomial of Back-operator for Time Series Models
-
AR型非线性时间序列模型的稳定性分析
Stability Analysis of AR-type Nonlinear Time Series Models
-
提出了DNA序列的时间序列模型,给出了DNA序列整数序列的一个映射。
A time series model of DNA sequence is proposed . A map of DNA sequence to integer sequence is given out .
-
重点介绍的是季节时间序列模型和处理异方差的自回归异方差模型(ARCH)。
The key of the thesis is seasonal time-series model and the ARCH model dealt with conditional variance .
-
在方法上,本文介绍了目前适合建立汇率波动预测模型的两种时间序列模型&ARMA模型和ARCH模型。
This paper introduces two time-series models-ARMA model and ARCH model , which are adapt to the fluctuation forecast .
-
文中着重分析比较了以下两种带宽预测算法:(1)AR时间序列模型;
We emphatically analyze two kinds of bandwidth prediction : ( 1 ) Autoregressive ( AR ) model ;
-
基于调查自内蒙古土默特平原的原始数据,编写SAS语言程序,建立了多元时间序列模型。
We established the time series model by writing SAS program and using original data .
-
应用拉氏变换和Z变换间关系,得到系统的ARMA时间序列模型。
By the relationship of Laplace transform and Z-transform , the ARMA time series model for this system is obtained .
-
利率构成中预期和未预期部分的分解,可以采用线性时间序列模型:ARMA模型,对其进行刻画。
We can use linear time series model such as ARMA model for decomposition of interest rates .
-
GAR模型为应用很广的一类非线性时间序列模型,AR模型为其特殊情况。
GAR models arc the general non-linear models and All model is its special case .
-
当然,为了进行比较,本人又用时间序列模型对原数据重新进行了短期预测,从两种模型的短期预测比较中就可以看出采用BP神经网络模型预测更精确,效果更佳。
Certainly the author forecast original data again using time series model in order to contrast and make a conclusion that the method using BP neural network is better .
-
Robinson(1994)检验被广泛应用于各种时间序列模型中。
The widely used Robinson test ( 1994 ) is applied to various well-known long memory models .
-
AR模型作为时间序列模型的一种,由于其参数估计和定阶简单而广泛用于系统辨识。
AR series , as one of time series models , is applied broadly in system identification because its parameter estimation and rank decision are simple .
-
作者在对石英音叉陀螺零点漂移数据建立时间序列模型(AR模型)的基础上,采用卡尔曼滤波算法对石英音叉陀螺的漂移数据进行了处理。
Based on the building of the time sequence model of MEMS gyro drift data , the MEMS gyro drift data is processed using Kalman filter .
-
该方法采用AR时间序列模型,预测故障后发电机转子角的变化趋势,并根据所构造的转子角预测模型多项式是否存在极值作为暂稳的识别判据。
By using AR model , the variation trend of generator rotor power angle can be predicted and the transient stability of power system can be determined .
-
交通流预测的研究模型有很多种,如:神经网络模型、多元线性回归模型、时间序列模型、历史趋势模型、kalman滤波模型等。
For example , multiple linear regression model , time series model , neural network model , the historical trend model , Kalman filter model and so on .
-
本文采用改进型BP神经网络建立起交通流的时间序列模型,该模型可用于短期内道路交通流量的预测。
In this paper , the time-sequence model of traffic flow is based on the improved BP neural network , and this model can be used for short time prediction of traffic flow .
-
而传统线性时间序列模型(如AR(p)或ARMA(p,q))无法刻画上述经济变量运行所表现出的多样性和不对称性的特征。
However , traditional linear time series models ( such as AR ( p ) / ARMA ( p , q )) can not describe the properties of diversity and asymmetry of above economic behavior .
-
对一类变量可分离时间序列模型的输入变量进行分解并构建基于RBF的分工协作模型,该模型对阶数较高的时间序列具有很好的逼近能力。
In this model the input of a variable-separable time series is decomposed . The divide-and-cooperate forecasting model is of excellent approximation ability in the time series with high order . 3 .
-
先将自相关过程的时间序列模型转化为状态空间模型,然后讨论了SPC与工程过程控制(EPC)的整合。
Time series model of processes is transformed into state space model . The concept of integrating SPC and engineering process control ( EPC ) have been discussed .
-
文章利用时间序列模型等计量方法,对中国GDP总量序列进行了时间趋势分解并结合实证结果对中国经济的发展周期进行了重新的划分。
Making use of time series model and other econometric approach , this article partitions the trend of China 's GDP data , and repartitions China 's economic cycle on the grounds of the empirical results .
-
本文通过AR1MA时间序列模型,利用SPSS统计软件对某电信产品收入进行了预测研究。
This worked out forecasting study on certain telecom products by SPSS statistic software with AR1MA time series model .
-
结论AR(2)-EGARCH(0,2)模型为本研究获得的预测效果较好的时间序列模型,适合于类似时间序列数据的结果预测。
Conclusion The established AR ( 2 ) - EGARCH ( 0,2 ) model is a time series model showed satisfactory forecasting ability in this study and is suitable for the forecasting of similar time series .
-
应用ARIMA时间序列模型预测临床用血量是可行的方法,可为采供血单位制定工作计划提供较为准确理论依据。
It is feasible to apply the model of ARIMA to predict the blood use in the future , and scientific basis will be provided in work plan decision .
-
采用Box-Jenkins非平稳时间序列模型,对码头的变形趋势进行了中、短期时间预测。
The model of non-stable time series raised by Box-Jenkins is applied to give a mathematical prediction of the deformation tendency .
-
利用实例仿真验证表明,LS-SVM具有较好的泛化能力和很强的鲁棒性,采用基于LS-SVM的交通流时间序列模型补齐丢失数据能够取得很好的效果。
The model for filling time series data of traffic flow based on LS-SVM is proposed in this paper , missing data can be filled by using traffic flow historical data .
-
根据秦沈客运专线高速试验段轨检车资料,采用ARMA时间序列模型模拟了高速铁路轨道不平顺随机样本函数;
With the data obtained from track geometry inspection car on Qinhuangdao-Shenyang special line for passenger transport and ARMA time series model , the sample function of high-speed railway track random geometric irregularity are simulated .
-
综合来看,本文采用的剔除食品的核心通货膨胀方法有一定的平抑波动,它反应长期趋势的作用,而由SAS拟合的时间序列模型效果也达到了不错的效果。
Taken together , this paper adopts the method of core inflation excluding food , have some stabilizing fluctuations , it reflects the role of long-term trends , but by SAS fitting time-series model results also reached good results .
-
文章运用时间序列模型描述了市场风险和特质风险的波动变化规律;运用GARCH系列模型专门实证分析了特质风险的动态变化和波动效应。
The paper described the risk characteristics of market volatility and idiosyncratic risk with using the time series models ; and empirically analyzed the dynamic changes and volatility effects of idiosyncratic risk with GARCH models .
-
提出了GA预测的方案,探讨了神经网络GA预测、时间序列模型GA预测,以及灰色模型GA预测的适合度选择,提供了进行遗传算法预测的操作步骤。
A plan of GA prediction was put forward . The ways for selecting fitness to the neural network GA predicting , the time series model GA predicting and the grey model GA predicting were probed . The operating steps of GA predicting have been provided .