高斯过程
- 网络Gaussian process
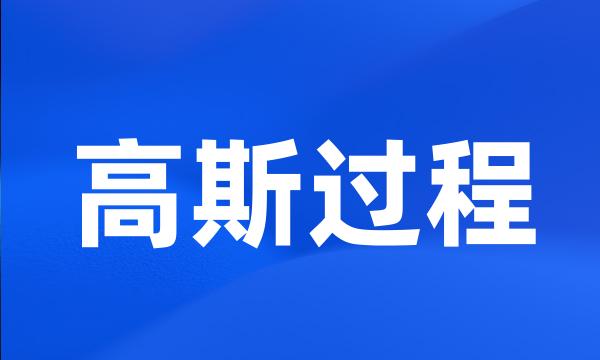
-
d维平稳高斯过程多重时的Hausdorff维数及Packing维数
Hausdorff dimension and packing dimension of multiple time set of d-Dimension stationary Gaussian processes
-
关于平衡高斯过程的Poincaré不等式和log-Sobolev不等式
Poincar é Inequality and Log-Sobolev Inequality for Stationary Gaussian Processes
-
利用GPS探测电离层异常中的高斯过程处理方法
Gaussian Random Process and its Application for Detecting the Ionospheric Disturbances Using GPS
-
将理赔到达过程推广为更新过程、广义复合Poisson过程、Cox过程、Gamma过程和逆高斯过程等等。
Under the assumption that the claim-arrival process is the renewal process , Cox process , generalized compound Poisson process , Gamma process and inverse Gaussian process etc.
-
假设基带OFDM信号为一带限复高斯过程,研究了带限OFDM信号峰平功率比(PAPR)的分布。
Assuming that the baseband OFDM signal is characterized by a band-limited complex Gaussian process , the distribution of the peak-to-average power ratio ( PAPR ) in band-limited OFDM signals is investigated .
-
在修复的结果上进行后续处理,使之看起来更真实?认为海浪是零均值、平稳、各态历经的高斯过程,波高谱服从P-M分布。
Gaussian smooth . The ocean waves are considered to be zero mean , stationary and ergodic gaussian random processes specified by the P-M wave height spectrum .
-
研究了满足Berman条件的局部平稳高斯过程{X(t),0≤t≤T}的最大值与最小值的联合渐近分布。
Let X ( t ), 0 ≤ t ≤ T be locally stationary Gaussian process that satisfies Berman ' condition . We study the asymptotic joint distribution of maxima and minima .
-
依据平稳随机过程&高斯过程的相关性质,利用其自协方差函数和TEC时间系列,构建了独立同标准正态分布的观测样本,并利用x~2假设检验的方法来探测电离层异常现象。
Based on this fact , here we made use of the time series of TEC and the auto-covariance function of the stationary process to construct independent identical distribution Gauss sample so that the X ~ 2 test can be used to detect the abnormity hidden in the sequence .
-
多参数平稳增量高斯过程的若干极限结果
Some Limit Results for the Multi-parameter Gaussian Processes with Stationary Increments
-
高斯过程的最大值的重对数律
An iterated logarithm law for the maximum in a Gaussian process
-
基于高斯过程机器学习的冲击地压危险性预测
Forecast of rock burst intensity based on Gaussian process machine learning
-
一种基于仿射传播聚类和高斯过程的多模型建模方法
Multi-model Modeling method Based on Affinity Propagation Clustering and Gaussian Processes
-
基于混合高斯过程的多模型热力参数测量软仪表
Thermal parameter soft sensor based on the mixture of Gaussian processes
-
离散非平稳高斯过程在实际中经常遇到。
The discrete nonstationary Gaussian processes are encountered in manypractical situations .
-
瓦斯涌出量预测的高斯过程机器学习模型
Forecasting Amount of Gas Emission Using Gaussian Process Machine Learning Model
-
用于高光谱遥感图像分类的空间约束高斯过程方法
A spatial Gaussian process method for hyperspectral remote sensing imagery classification
-
在混合条件下非平稳高斯过程的联合逗留极限定理
Limit laws for joint sojourns of Gaussian process under mixing conditions
-
具有高斯过程输入的一类非线性系统的输出相关函数
The output correlation function of some nonlinear systems with Gaussian process input
-
一类平稳高斯过程象集代数和的性质
Properties of Algebraical Sum of Image Sets of the Stationary Gaussian Process
-
d维平稳高斯过程的极函数及其相关维数
Polar Functions and Relative Dimension for d-Dimensional Stationary Gaussian Processes
-
零均值、窄带高斯过程的过阀特性
Statistical Property of Threshold-Crossing for Zero - Mean-Valued , Narrow-Banded Gaussian Processes
-
高斯过程机器学习在边坡稳定性评价中的应用
Application of Gaussian process machine learning to slope stability evaluation
-
基于高斯过程分类器的连续空间强化学习
Reinforcement Learning for Continuous Spaces Based on Gaussian Process Classifier
-
详细介绍了高斯过程的经典书籍,应用在机器学习上。
Detailed introduction of Gaussian Process modal applied in Machine learning Domain .
-
第二,提出了基于稀疏高斯过程的多用户检测技术。
Proposed the based on sparse Gaussian process multiuser detection .
-
关于平稳高斯过程的上穿过期望次数的几点注记
Notes on Mean Number of Upcrossings for Stationary Gaussian Process
-
非平稳高斯过程的最大值的渐近性质
Asymptotic properties OE the maximum in a nonstationary Gaussian process
-
d维平稳高斯过程极集的必要条件
The Necessary Conditions of the Polar Sets for d-Dimension Stationary Gaussian Process
-
d维平稳高斯过程相交局部时的几个性质
Several Properties of Joint Local Time of d Dimension Stationary Gaussian Processes
-
高斯过程是最重要的随机过程。
Gaussian process is the most important random process .