维数灾难
- 网络Dimensionality Curse;The Curse of Dimensionality
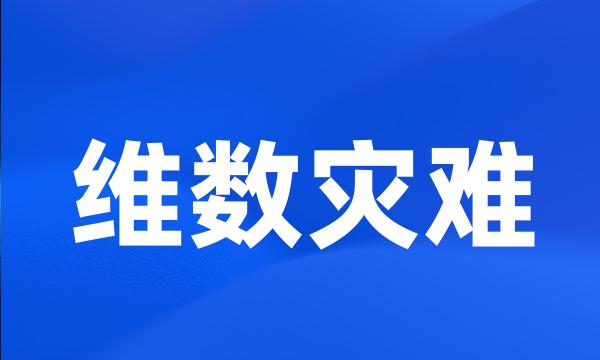
-
该方法把经典的基于相关的DNA微阵列数据聚类分析方法和图论知识相结合,能更好地揭示基因或样本之间的相似性,且避免了维数灾难问题。
It can penetrate into the similarity between tissues or genes , while avoiding the problem of curse of dimensionality .
-
此算法利用Apriori算法的搜索技术来发现强模糊规则,因此具有较高的算法效率,并有效地解决了模糊系统的维数灾难问题。
The fast algorithm uses search technology of Apriori algorithm to discover strong fuzzy rules , so it has high efficiency and solves effectively the curse of dimensionality in the fuzzy systems .
-
该现象被称为维数灾难(dimensionalitycurse),主要原因是存在数据重复。
This phenomenon is called dimensionality curse .
-
支持向量机(SVM)可以优化网络,有效降低模型复杂性,不存在维数灾难和局部极小问题。
SVM can optimally create network structure automatically , efficiently reduce the modeling complexity , and without local minima , dimension disaster .
-
针对已有经典方法在大规模参数优化中存在维数灾难等问题,本文将微粒群算法与模糊C均值聚类相结合,提出动态多种群协同微粒群优化方法。
To solve the problem of " curse of dimensionality " faced by traditional optimal methods , the particle swarm optimization algorithm and fuzzy C-means clustering method are combined to complete multi-group cooperative particle swarm optimization algorithm .
-
该方法为克服维数灾难,实现在线辨识Volterra级数提供了一个有效解决途径。最后,文中用一个工程实例验证了该算法的有效性。
This method is suitable for Volterra identification on line and its effectiveness has been validated by an engineering instance .
-
本文提出了一种新的非线性系统Volterra级数模型辨识方法,为非线性系统辨识中的维数灾难问题提供了一种满意的解决。
A new algorithm of nonlinear system identification for Volterra series model is presented in this paper . The algorithm provides a satisfactory solution to the dimension disaster of nonlinear system identification .
-
最后基于神经网络学习的benchmark问题对各种算法在网络训练中的应用性能进行了仿真研究,并提出了遗传算法受困于维数灾难的观点。
Finally the training performances of various algorithms are compared based on a simulation experiment on a benchmark problem of neural network learning , furthermore , a viewpoint that genetic algorithm is subject to " curse of dimension " is proposed .
-
基于统计学的最近邻查询中维数灾难的研究
Study on Dimensionality Curse in the Nearest Neighbor Queries Based on Statistics
-
此方法能解决维数灾难问题。
This method can overcome the curse of dimensionality .
-
强化学习的维数灾难一直是其在路径规划问题中难以克服的问题。
The dimension disaster is always a big problem of reinforcement learning for path planning .
-
它作为克服维数灾难的途径在这些相关领域中扮演着重要的角色。
It as to overcome " dimension disaster " approach in the related field plays an important role .
-
为了避免维数灾难问题,需要对高维数据进行特征降维。
Then it is necessary to reduce the dimension of the data sets to avoid the curse of dimensionality .
-
这种模糊神经网络可以根据样本自动产生模糊规则,在一定程度上可避免维数灾难。
According to sample , this kind of fuzzy neural network can generate fuzzy rules automatically and can avoid " dimensional disaster " to some extent .
-
现有辨识算法仍存在一些难题,例如避免维数灾难和提高模型泛化能力的问题。
However , the existing identification algorithm is still facing the problems of how to avoid the curse of dimensionality and improve the model generalization capability .
-
如何克服高维连续空间带来的维数灾难,实现增强学习算法在连续空间中的泛化,是增强学习进一步发展并向工程应用领域推广的关键,是本文的主要研究内容之一。
How to conquer the curse of dimensionality and achieve good generalization performance in continuous space is significant for the future development and application of reinforcement learning .
-
为了解决这种维数灾难问题,研究者引入了分层强化学习的概念并提出了一些有效的方法。
To avoid the so-called ' curses of dimensionality ', some methods have been put forward , HRL ( hierarchical reinforcement learning ) was among of them .
-
信息的过量繁杂,有效信息隐藏在海量信息中难以发觉;而且更为严重的是,现有的机器学习和数据挖掘算法难以对其进行有效处理,直接导致了维数灾难的产生。
And more serious is that the existing machine learning and data mining algorithms can hardly deal with them effectively , which leads the " Dimension Disaster " .
-
在机器学习和模式识别领域,会不可避免地遇到很多高维数据,从而出现维数灾难。
In machine learning and pattern recognition , it is inevitable to encounter with high-dimensional data sets , which usually cause a problem called the curse of dimensionality .
-
随着高维数据的急剧涌现,模式识别和机器学习领域面临着维数灾难问题的严峻挑战。
With the rapid emergence of high-dimensional data , the so-called ' curse of dimensionality ' problem has become the sever challenge in pattern recognition and machine learning .
-
随着电力系统规模越来越庞大,传统的最优潮流计算呈现出计算机内存不足,收敛速度慢等维数灾难问题,不能满足实时性要求。
Along with the enlarging of electric network , the problems which longtime computing and slow rapidity of convergence become serious in the computing of traditional optimal power flow .
-
内容检测时提取的特征向量往往具有高维特性,传统的多维索引结构在高维空间中会面临着维数灾难问题。
The dimensionality of feature vectors extracted from the multimedia objects is often very high , when traditional indexing methods face the difficulty of ' curse of dimensionality ' .
-
大量无关或冗余特征的存在不仅造成了维数灾难,还直接影响了分类器性能,因此需要对数据进行特征选择。
Too many irrelevant and redundant features will not only cause the curse of dimensionality , but impact the performance of classifiers directly , so feature selection is important .
-
光滑参数的选取、“维数灾难”与边界点问题也是与非参数技术有关的重要内容。
The estimation of the smooth parameter , the problem of " the curse of the dimension " and the boundary are also important matter in the nonparametric technique .
-
然而,在大状态空间,特别是在复杂随机状态下的应用领域,它仍然没有解决“维数灾难”的问题。
However , in the large state space , especial for application problems with complex stochastic states , the " dimension curse " problem hasn 't been solved yet .
-
目前,图像检索面临的一个重大问题仍是维数灾难,利用特征选择来进行维数约简是图像检索过程的必要环节。
Currently , what the content based image retrieval is facing is the dimensionality disaster . It is an indispensable step to make dimensionality reduction through the feature selection .
-
该算法包含3个重要的方面:通过在算法迭代过程中对松弛后的0、1变量与机组有功出力变量并行地进行优化,避免了由于决策变量过多造成的维数灾难题;
The new method includes three important aspects : to employ parallel optimization of relaxed zero-one variable and the active power continuous variable to overcome the curse of dimensionality problem ;
-
为解决传统索引方法对高维数据索引时存在的维数灾难问题,提出一种多分辨率向量近似方法。
In order to reduce the curse of dimensionality faced by the traditional indexing method at high dimensionality , a new MRVA-File ( Multi-Resolution Vector Approximation File ) approach is proposed .
-
适合于小样本的学习,具有很强的泛化性能,不会出现其他预测模型难以解决的局部极值和维数灾难等问题。
Suitable for small sample study , has a strong generalization performance , the other prediction models will be difficult to resolve the problems of local minima and the curse of dimensionality .
-
在机器学习、模式识别、信息检索和生物信息等很多领域人们都面临海量的高维数据,由此引发维数灾难问题。
In many areas of machine learning , pattern recognition , information retrieval and bioinformatics , one is often confronted with the massive high-dimensional dataset , which leads to the curse of dimensionality .