chmm
- 网络连续隐马尔可夫模型;连续隐马尔可夫;连续型隐马尔可夫模型
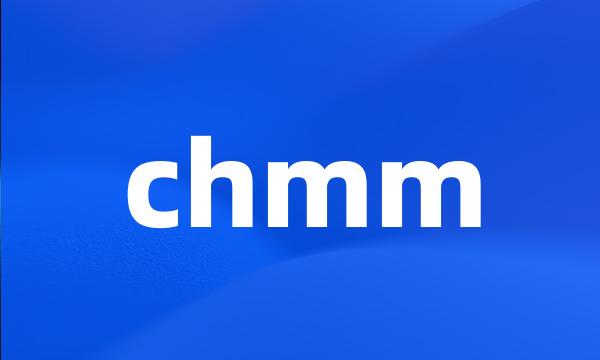
-
A Chinese Mobile Input Method Based on CHMM and Its Implementation
基于CHMM模型的手机中文输入方案及实现
-
New Speech Recognition Method Based on Genetic Algorithm Training CHMM
利用基因算法训练连续隐马尔柯夫模型的语音识别
-
Digital Speech Recognition System Based on Hybrid Model of CHMM and MLP
基于混合模型CHMM和MLP的数码语音识别系统
-
Research on CHMM Based Equipment Performance Degradation Assessment
基于CHMM的设备性能退化评估方法研究
-
A Study of Connected Digit Speech Recognition Based CHMM with Prosodic Information
利用韵律信息的CHMM连续数字语音识别
-
Fast implementation of isolated-word recognition system based on CHMM
基于CHMM孤立词识别系统的快速实现
-
Investigation on Speech Emotion Analyses and Its Application in Education Based on MFCC and CHMM Techniques
基于MFCC和CHMM技术的语音情感分析及其在教育中的应用研究
-
Conclusion : CHMM has immune regulatory effect on cell mediated immune function in patients of burn .
结论:中药合剂对烧伤患者细胞介导的免疫功能有调理作用。
-
Automatic audio classification based on PCA and CHMM
基于PCA和CHMM的音频自动分类
-
At present , advanced high performance speech recognition system is usually based on Continuous Hidden Markov Model ( CHMM ) .
目前高性能的语音识别系统,都是基于连续隐含马尔可夫模型(CHMM)的。
-
The research of PDT to exosomatic lethal effect of canine breast tumor cells lines CHMm
PDT对犬乳腺肿瘤细胞株CHMm的体外杀伤效应的研究
-
A CHMM speech recognition using commixing high-dimensional diagonal covariance
基于统合高维对角协方差的CHMM语音识别
-
When the feature of pattern and the station of model are defined , the complexity of CHMM mainly refers to the number of Gauss distributions .
结论指出,实现CHMM模型结构的优化设计包括对训练精度和复杂度的优化选择,在特征和状态确定时,模型的复杂度主要是混合Gauss数。
-
Furthermore , it is pointed out that the structure optimization of CHMM consists of how to choose the precision and the complexity appropriate to the number of available training samples .
在训练样本数一定的情况下,模型结构的复杂度和训练精度存在最优解。
-
To recognize isolated words in noisy environments , A new robust recognition model with dual recognition procedures based on continuous Hidden Markov Models ( CHMM ) and BP neural networks ( BPNN ) is presented .
论文提出了一种连续隐Markov模型和BP神经网络相结合的、具有两次辨识过程的抗噪孤立字识别模型。
-
A modified CHMM model is proposed to improve the robustness of the speaker recognition system . In the modified model , the difference of the speech feature parameter was the observed vectors of the state transitions of CHMM .
为了提高话者识别系统的噪声鲁棒性,本文对CHMM进行了改进,将每帧特征参数之间的差分参数来对应状态之间的转移,从而使帧间信息在模型中得到了体现。
-
For the reason of the theory of human aural perception , in this paper , we present a system based on the fusion of multi-band Continuous HMM ( CHMM ) and Back Propagation Neural Network ( BPNN ) .
根据人类的听觉感知机理,本文提出了一种改进的基于多带连续隐马尔科夫(CHMM)和BP神经网络融合的识别算法。
-
I implement the parametric stochastic trajectory model in text-dependent speaker recognition ; and because of the strong ability in exploiting the time correlation of speech frames , the result is superior to those attained by GMM and CHMM . 3 .
参数化随机轨线模型具有很强的挖掘连续语音帧之间的时序性的能力,实现其在文本相关的说话人识别中的应用,效果要好于GMM和HMM。
-
CHMM is built by using 12 rank LPC cep-strum coefficient to extract feature vectors , scaled for-wards-backwards algorithm is introduced to calculate log-likelihood avoiding the data to underflow and k-means algorithm is also used to initialize the parameter .
采用12阶LPC倒谱系数进行特征提取,建立CHMM,为防止数据下溢,引入前向一后向比例因子算法求其对数似然概率,并且采用K-means算法对CHMM进行参数初始化。
-
We discuss how to choose proper CHMM state numbers by simulation and provide a group of CHMM for OFDM system in HIPERLAN / 2 indoor wireless channel , which can be used to facilitate the comparison of different coding schemes and analysis of high-level protocol performance .
通过仿真讨论了CHMM状态数的选取问题,并给出了HIPERLAN/2室内无线信道环境下OFDM系统的一组CHMM,该模型可用于比较不同编码方案的性能或者分析高层协议性能。
-
According to this algorithm , the output from CHMM is taken as the feature vector for recognition and the pre-recognition vector is extracted from the recognition vector space by the ANN , afterwards , the pre-recognition result is further processed to accomplish the final recognition .
该方法以CHMM的输出作为系统的识别矢量,利用人工神经网络的模式分类和自学习功能,从识别矢量空间中提取语音预识别矢量,再由识别矢量对预识别结果进行识别输出。