Multicollinearity
- 网络多重共线性
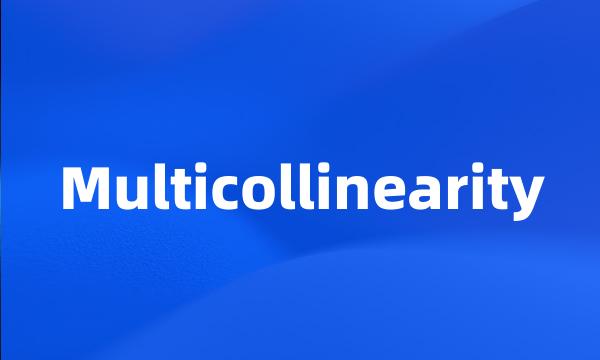
-
Research on the Multicollinearity Existing in Observation Data Simulation and Analysis
观测数据拟合分析中的多重共线性问题
-
Via factor analysis , the multicollinearity problem is avoided . 2 .
通过因子分析,避免了后续回归分析中存在的多重共线性问题。
-
Implementation of Mining Algorithm for the Regression Model Based on Spatial Multicollinearity Data
复共线性空间数据回归模型挖掘算法及其实现
-
VAR model has good forecast effect and Stepwise regression can solve multicollinearity .
Grange因果关系检验、VAR模型被证明具有较好的预测效果;逐步回归则有效的克服了多重共线性带来的问题。
-
Firstly , we analyze the multicollinearity among features to eliminate redundant features .
首先,通过分析特征间的多重共线性去除冗余特征。
-
Conclusion : This method has the practically applied value in resolving the multicollinearity .
结论:在解决共线性的问题中有实际应用价值。
-
A new class of way to combat Multicollinearity
克服复共线性的一种新方法
-
Partial Least Square Regression can resolve the problem of multicollinearity among variables .
偏最小二乘回归能较好地解决自变量间的多重共线问题,最小一乘法比最小二乘法能更有效地降低回归模型的误差。
-
The relation between ill conditioning and multicollinearity is discussed .
探讨了病态性的概念及其与复共线性的关系;
-
Properly selecting parameters to avoid stronger multicollinearity is a key to efficient estimation of parameters .
合理地选择参数,避免参数间的强复共线性,是参数有效估计的关键之一。
-
Biased estimator based on diagnosis and measure of Multicollinearity
基于复共线性诊断和度量的有偏估计
-
The parameter estimation problem in linear model is considered when multicollinearity and outliers exist simultaneously .
本文讨论复共线性和粗差同时存在时线性模型的参数估计问题。
-
The study of the multicollinearity in animal traits
动物性状中多重共线性初探
-
The least Square estimates are not reliable when there exists multicollinearity in adjustment model .
当平差模型中存在复共线关系时,未知参数的最小二乘估计很不可靠。
-
Pseudo Adaptation Data ( PAD ) method was proposed for decreasing the degree of multicollinearity .
为减轻多重共线性的影响,提出改进算法:伪自适应数据算法。
-
Then , we conducted a correlation analysis found that this variable does not exist a serious multicollinearity problem .
然后,本文进行了相关性分析,发现本文所涉及的变量不存在严重的多重共线性问题。
-
However , when multicollinearity between variables , the least squares estimate will be limited .
然而,当变量间存在复共线性问题时,最小二乘估计就会有所限制。
-
Amelioration of Common Ridge Estimates Under the Condition of Multicollinearity
复共线条件下普通岭估计的改进
-
Conclusion The new method is effective and feasible for diagnosis and treatment of multivariable multicollinearity in the logistic regression model analysis .
结论在Logistic回归模型分析中应用上述方法进行多重共线性的诊断和处理是有效及可行的。
-
The essay has mainly studied about two aspect content : The recognitions and revision of multicollinearity in linear model ;
本论文主要研究了以下两个方面的内容:线性模型中多重共线性的识别与修正;
-
Especially , the advantages of the method are marked , while the variables Xs multicollinearity being serious .
特别,当变量X多重相关性突出时,本文方法效果显著。
-
The paper discusses two questions : one about multicollinearity , and the other about the weakness of Partial Least Square Regression .
文章探讨了两个问题,一个是关于复共线问题,另一个是关于偏最小二乘回归的弱点问题。
-
Multicollinearity and Its consequence in Multiple Regression
多元回归中的多重共线性及其存在的后果
-
The coefficients of variables in the model are statistically significant , and the multicollinearity is proved to be weak .
数学模型中各变量系数在统计意义上显著,且各系数无明显多重共线性。
-
Like the least squares estimate , the restricted least squares estimate is also not good to deal with the multicollinearity .
经过研究发现,约束最小二乘估计同最小二乘估计一样,在处理共线性问题上也存在着不足。
-
The staple methods of elimination multicollinearity and betterment methods of parameters are principal component regression and ridge regression .
消除多重共线性常用的参数改进方法有主成分回归和岭回归。
-
Results : The analyses of two examples illustrate that leverage points or influential points may enhance or mask the effect of multicollinearity .
结果:通过两个实例分析说明数据中的杠杆点或影响点可夸大或掩盖多元共线性。
-
Among the problems of existing models , the problem of selecting of prediction parameters and the problem of multicollinearity have never being solved effectively .
目前在模型建立上仍然存在着一些问题,如:预测变量的选择、多重共线性等问题,始终没有得到有效的解决。
-
On the analysis of the correlation between variables is investigated the correlation between each variable and the existence of selected variable multicollinearity problems .
对变量之间进行相关性分析,考察所选变量是否存在多重共线性问题。
-
In the light of the approximate multicollinearity of matrix , distance for principal components estimation ( namely distance ) is put forward .
针对设计矩阵的多重共线性问题,为了改进基于最小二乘估计的统计诊断量Cook距离,提出了基于Massy主成分下的Cook距离(MPCC距离)。